Health is determined by many factors including:
- Behavior (Physical Activity, Eating habits, Tobacco or substance abuse, responsible sexual choices, etc)
- Mental Health
- Injury and Violence
- Environmental Quality
- Preventative measures such as immunization
- Access to Health Care
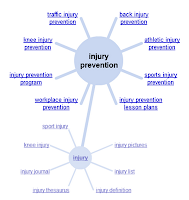
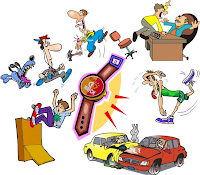
There are many ways to prevent injuries - just say "no" to risky behaviors, wear preventative gear while playing sports or fall-optimized shoes for elderly, watch out for others engaged in similar activities... Yet, sometimes we forget to watch, don't have access to histories of others or get diverted. Would a body sensor or a gadget with smart software be able to warn us about potential accidents ahead to help prevent accidents? What would it need to measure?
Software and devices automatically detecting and reporting accidents already exist:
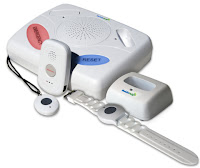
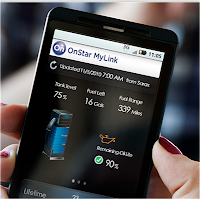
sensors, heart rate and skin temperature monitors. Although the system detects falls only after they happen, a study showed that just the fact of wearing it increases alertness of seniors and reduces the number of falls. Although fall detection systems are not as advanced as telematics for cars - like
In the always-connected smart-sensor-equipped future, things such as Intel's magic carpet - picking up the weight, angle and pressure of steps - will be a commodity. Gene tests predicting injuries will be integrated with data coming from our carpets, clothing, footwear and location information. And this may be sooner than you think.
References
1. Centers for Disease Control and Prevention (CDC), National Center for Injury Prevention and Control. Web-based Injury Statistics Query and Reporting System (WISQARS); 2010 Mar 4 Available from: http://www.cdc.gov/injury/wisqars/index.html
2. Husted JA, Ahmed R, Chow EW, Brzustowicz LM, Bassett AS. Childhood trauma and genetic factors in familial schizophrenia associated with the NOS1AP gene. Schizophr Res. 2010 Aug;121(1-3):187-92. PMID: 20541371
3. Swanenburg J, de Bruin ED, Uebelhart D, & Mulder T (2010). Falls prediction in elderly people: a 1-year prospective study. Gait & posture, 31 (3), 317-21 PMID: 20047833
3. Swanenburg J, de Bruin ED, Uebelhart D, & Mulder T (2010). Falls prediction in elderly people: a 1-year prospective study. Gait & posture, 31 (3), 317-21 PMID: 20047833
4. Kononen DW, Flannagan CA, Wang SC. Identification and validation of a logistic regression model for predicting serious injuries associated with motor vehicle crashes. Accid Anal Prev. 2011 Jan;43(1):112-22. PMID: 2109430
5. Price GR. Predicting mechanical damage to the organ of Corti. Hear Res. 2007 Apr;226(1-2):5-13. Epub 2006 Sep 15.PMID: 16978813