The title for the session was "Biomedical Data Mining: Successes, Failures, and Challenges" (streamed online from Fireside C).
The topic stemmed from the similarly named last year's session - Biomedical Data Mining: Dimensionality, Noise, Applications - now split into several discussions including Bioinformatics & Genome Sequencing organized by Raymond McCauley, Dimensionality Reduction moderated by Luca Rigazio (HLDA / HDA; LDPP; Core Vector Machines; Sparse Proj SQ; Random Projection and Feature Selection).
Main sub-topics of the biomedical session were:
- Reality Mining
- Visualization
- Imaging
- Signal Processing
Another interesting aspect of reality mining is crowdsourcing or collective intelligence - in order to get useful information from all the data (temporospatial location, GPS, activity, food, symptoms, behavior, communication content, proximity sensing), we need to analyze it not only on individual but also group level. We need to share more, without sacrificing privacy and security. Collective contributions can be reliable - Shamod Lacoui's answer to this is in selecting those who contribute, restricting inputs to domain. It would help to “filter out the dross”, while “saving the best”. It is needed to suppress noise, to infer intelligence from the collection of facts, clicks, steps, whatever one can contribute. This resonates with discussions at the Transparency Camp - one of the useful tools is SwiftRiver - free, open source software platform to validate and filter news. Swift relies on Natural Language Processing, Machine Learning and Veracity Algorithms to track and verify the accuracy of reports and suppress noise (like duplicate content, irrelevant cross-chatter and inaccuracies). Transparency Camp also posed a question on whether there is a need for an FDA-like institution to ensure information safety and healthy information consumption.
Self-serve was a topic of a smaller Data Mining Camp Session. Even though it was aimed at sales reps that need to go beyond Excel spreadsheets to mine private data of their interest, self-service is currently the only option for health care consumers. People need to analyze everyday life for health implications. They need better tools to not focus on metrics that are easy to collect instead of metrics we need to collect.
In order to mine high-dimensional health space, many disparate types of data should be mashed and validated, gaps should be bridged and structured metadata added to data. Randy Kerber talked about data formats and approaches to make it happen. Semantic web discussions involved NoSQL experts that mentioned limitations of gaining popularity technologies such as MongoDB, Cassandra and HBase. Another relevant session - on cloud computing - discussed its (sometimes over-rated ?) performance and Hadoop technologies.
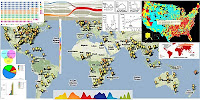
One of the participants of Biomedical Session developed kidsdata.org (@kidsdata on twitter). It provides insights into geospatial autism statistics and visualizes trends and other useful health-related information.
Another way to display geospatial data is Dynamic Choropleth Maps. Complex networks can be also explored with alluvial diagrams and other approaches. More visualization techniques and tools can be applied to health data - to look at the data in new ways and gain useful insights.
Some of the questions from the audience were on the availability of data. Sources discussed included CDC (see, for example, NHANES laboratory files; eHealth metrics) and Entrez Life Sciences databases.
Signal Detection and Signal Processing for Mining Information was another discussion topic.
Questions were on data mining versus simple tracking and signal monitoring. It was agreed that data mining is the key to health management. Cardionet, body sensors (see posts on teletracking, M-health, Telemedicine: part 1; Telemedicine: part 2; Health 2.0 Software tools, Devices to keep you healthy), SNP detection, telemedicine applications, random and rare electrocardiographic events and other applications were also discussed.
See other materials from Data mining Camp 2010:
- ACM Data Mining Camp, March 20, 2010 (sfbayacm.org)
- Data Mining Camp Report (Zemanta)
- Mining Data mining Camp Impressions (Aurametrix)
- My Experience at ACM Data Mining Camp #DMcamp (bytemining.com)
- Transparency Camp, March 27-28, 2010 (The Sunlight Foundation)
- Notes from Transparency Camp (David “Oso”, Rising Voices)